Path following vs Trajectory Tracking MPC¶
Introduction¶
In this example we will see how to define trajectory tracking and path following control problems using Neo.
For this example we consider a point mass. The goal is to follow this shape by using a path-following approach.
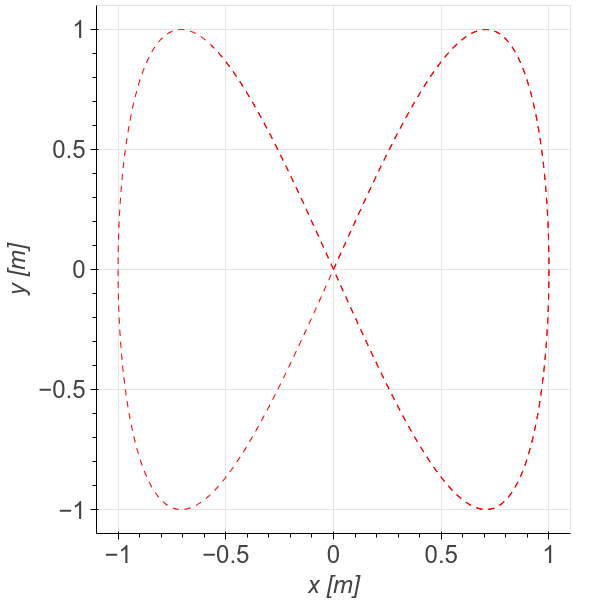
the equation that describe it are \(x(\theta) = sin(\theta)\) and \(y(\theta) = sin(2 \theta)\).
First import the necessary packages
[1]:
# Add NMPC to path. NOT NECESSARY if it was installed via pip.
import sys
sys.path.append('../../../')
# Import NMPC and Model classes
from hilo_mpc import NMPC, Model
import casadi as ca
Build the model¶
[2]:
# Define the model
model = Model(plot_backend='bokeh')
# Constants
M = 5.
# States and algebraic variables
xx = model.set_dynamical_states(['x', 'vx', 'y', 'vy'])
model.set_measurements(['y_x', 'y_vx', 'y_y', 'y_vy'])
model.set_measurement_equations([xx[0], xx[1], xx[2], xx[3]])
x = xx[0]
vx = xx[1]
y = xx[2]
vy = xx[3]
# Inputs
F = model.set_inputs(['Fx', 'Fy'])
Fx = F[0]
Fy = F[1]
# ODEs
dd1 = vx
dd2 = Fx / M
dd3 = vy
dd4 = Fy / M
model.set_dynamical_equations([dd1, dd2, dd3, dd4])
# Initial conditions
x0 = [0, 0, 0, 0]
u0 = [0., 0.]
# time interval
dt = 0.1
model.setup(dt=dt)
Path-following problem¶
Once the model is setup, we can pass it to the path-following MPC. Defining a path-following problem can be done in 3 steps:
Run
create_path_variable()
to generate the path variableDefine the path function using the path variable
Pass the path function function to the
ref
argument of stage and terminal cost and putpath_following=True
NOTE: you can create only as many path variables and you can have as many different path functions as you want.
[3]:
# Define path following MPC
nmpc = NMPC(model)
theta = nmpc.create_path_variable(u_pf_lb=1e-6)
nmpc.quad_stage_cost.add_states(names=['x', 'y'], weights=[10, 10],
ref=ca.vertcat(ca.sin(theta), ca.sin(2 * theta)), path_following=True)
nmpc.quad_terminal_cost.add_states(names=['x', 'y'], weights=[10, 10],
ref=ca.vertcat(ca.sin(theta), ca.sin(2 * theta)), path_following=True)
nmpc.horizon = 10
nmpc.set_initial_guess(x_guess=x0, u_guess=u0)
nmpc.setup(options={'print_level': 0})
Then run the control loop
[4]:
# Prepare and run closed loop
n_steps = 200
model.set_initial_conditions(x0=x0)
sol = model.solution
xt = x0.copy()
for step in range(n_steps):
u = nmpc.optimize(xt)
model.simulate(u=u)
xt = sol['x:f']
******************************************************************************
This program contains Ipopt, a library for large-scale nonlinear optimization.
Ipopt is released as open source code under the Eclipse Public License (EPL).
For more information visit http://projects.coin-or.org/Ipopt
******************************************************************************
The results look like
[5]:
from bokeh.io import output_notebook, show
from bokeh.plotting import figure, gridplot
import numpy as np
# define path function for plotting
def path(theta):
return np.sin(theta), np.sin(2 * theta)
x_path = []
y_path = []
for t in range(1000):
x_p, y_p = path(t / 100)
x_path.append(x_p)
y_path.append(y_p)
# Plot using bokeh
output_notebook()
p_pf = figure(title='Path following problem')
p_pf.line(x=np.array(model.solution['x']).squeeze(), y=np.array(model.solution['y']).squeeze())
p_pf.line(x=x_path, y=y_path, line_color='red', line_dash='dashed')
# p = format_figure(p)
p_pf.yaxis.axis_label = "y [m]"
p_pf.xaxis.axis_label = "x [m]"
show(p_pf)
Trajectory tracking problem¶
Trajectory tracking problems are formulated similarly to path following problem. For the case where the function of the trajectory is available, do as follows:
Run
create_time_variable()
to generate the time variableDefine the time-varying function using the time variable
Pass the time function function to the
ref
argument of stage and terminal cost and puttrajectory_tracking=True
NOTE: if the function of the reference is not available, you can pass directly the data points when solving the MPC. see the documentation for more info.
[6]:
# Define trajectory tracking MPC
nmpc = NMPC(model)
time = nmpc.get_time_variable()
nmpc.quad_stage_cost.add_states(names=['x', 'y'], weights=[10, 10],
ref=ca.vertcat(ca.sin(time), ca.sin(2 * time)), trajectory_tracking=True)
nmpc.quad_terminal_cost.add_states(names=['x', 'y'], weights=[10, 10],
ref=ca.vertcat(ca.sin(time), ca.sin(2 * time)), trajectory_tracking=True)
nmpc.horizon = 10
nmpc.set_initial_guess(x_guess=x0, u_guess=u0)
nmpc.setup(options={'print_level': 0})
The rest is the same
[7]:
# Prepare and run closed loop
n_steps = 100
# the solution stored in the model must be reset (initial conditions are kept by default)
model.reset_solution()
sol = model.solution
xt = x0.copy()
for step in range(n_steps):
u = nmpc.optimize(xt)
model.simulate(u=u)
xt = sol['x:f']
[8]:
x_path = []
y_path = []
for t in range(1000):
x_p, y_p = path(t / 100)
x_path.append(x_p)
y_path.append(y_p)
# plot using bokeh
output_notebook()
p_tt = figure(title='Trajectory tracking problem')
p_tt.line(x=np.array(model.solution['x']).squeeze(), y=np.array(model.solution['y']).squeeze())
p_tt.line(x=x_path, y=y_path, line_color='red', line_dash='dashed')
# p = format_figure(p)
p_tt.yaxis.axis_label = "y [m]"
p_tt.xaxis.axis_label = "x [m]"
show(p_tt)
Comparison¶
[9]:
from bokeh.models import Range1d
p_pf.x_range = Range1d(-1,-0.5)
p_pf.y_range = Range1d(0.5, 1)
p_tt.x_range = Range1d(-1,-0.5)
p_tt.y_range = Range1d(0.5, 1)
show(gridplot([p_pf,p_tt], ncols=2))